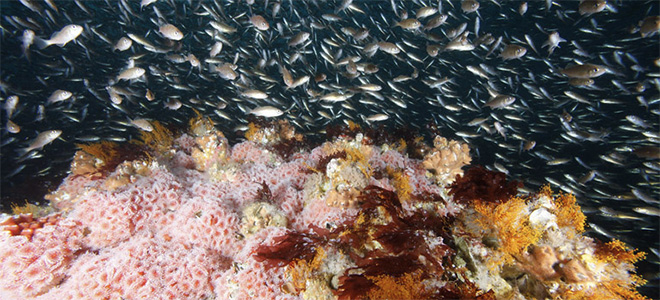
by Ernesto Carrella, E., S. Saul, K. Marshall, M.G. Burgess, R.B. Cabral, R.M. Bailey, C. Dorsett, M. Drexler, J. Koed Madsen, and A. Merkl
Ecological Economics, Volume 169 (2020)
Introduction: We calibrate and validate the POSEIDON (Bailey et al., 2018) fisheries agent-based model using data from the US West Coast groundfish fishery and compare the performance of simple, adaptive algorithms with imperfect information with other, more commonly used decision-making algorithms that include perfect information and/or rationality. We show that the adaptive algorithms explain observed data better. Moreover, while it is possible to derive statistical agents from logbook data, a simple, adaptive, uncalibrated decision-making algorithm performs out-of-sample just as accurately.
We address two gaps in the literature. First, we compare the usual assumption in bioeconomic models of allocating effort automatically where profits would be maximized (“assuming away the problem of finding fish”, Wilson, 1990) to more “bounded” rationality, either in terms of information available or ability to process it. Second, we implement multiple decision-making processes within the same bioeconomic model; these way decision-making algorithms can be compared not just by their ability to predict future actions but also on the system-wide effects they have over the biology and economic performance.
Two recent bioeconomic models focused on the US West Coast groundfish fishery. Toft et al. (2011) modelled groundfish trawlers as they entered the individual transferable quota (ITQ) program while Kaplan et al. (2014) modelled the effects of 20 fleets, representing gear types, on the whole California Current ecosystem. Both studies assume agents know perfectly the profits they will make in each area before making a trip.
More generally, Van Putten et al. (2012) classifies behavioural models of fishers into three groups: dynamic optimization, discrete-choice models or agent-based models. For all three it is rare to model exploration and learning directly; more common is to either assume “perfect knowledge”, agents knowing already the profits they will make before travelling, or rational expectations, agents having the correct expectations of what profits or catches will be.
Dynamic optimization fishers compute the optimal long term plan by allocating effort in time and space by value iteration (Clark and Mangel, 2000). Because of its computational complexity (see Littman et al., 1995) perfect knowledge is an important expedient to keep the problem dimension small and computable. Dynamic programming fishers in Dowling et al. (2012) not only know abundance throughout the ocean but also stock dynamics and migratory patterns. Similarly, the Alaskan multispecies groundfish trawlers in Ono et al. (2017) know the yields of all metiers, mixing them optimally through linear programming. Boettiger et al. (2015) manage to add uncertainty to a dynamic programming problem but to do so they remove geography and have only one representative fisher (which makes it hard to study allocative results of policies).
Expectations of catches or profits are a key component in discrete-choice models. These expectations can be the actual catches or profits the fisher will make or a noisy, lagged observation of them as in Mistiaen and Strand (2000). Fishers in Haynie and Layton (2010) know correctly the average catches they will make in each area as do the recreational fishers in Baerenklau and Provencher (2005). In the dynamic model of Hicks and Schnier (2006), fishers not only perfectly predict catches in every area but can mentally simulate their evolution through time and no new information can be obtained by either searching or fishing. Read more …